(With apologies to /r/WitchesVsPatriarchy)
Artificial intelligence is weird. You pull a thread on a tiny technical issue, and the problem swiftly unravels half the universe until you’re staring at a deep unsolved philosophical quandary. “What capabilities does a language model possess?” becomes “what capabilities does a human possess?” and then “what does ‘capable’ even mean?”
After all, many things are theoretically capable of stuff. A lottery ticket is capable of making you a millionaire, a thousand monkeys is capable of typing the works of Shakespeare, and paint randomly spraygunned over a canvas is capable of producing a Monet.
We don’t care about such “capability”, though, because we can’t reliably access it. A lottery ticket can make you a millionaire, but you’ll assuredly go broke buying them long before that happens. A thing that exhibits an ability once in a blue moon (or under some contrived set of circumstances) is of little practical use.
The real test of any artificial (or human) intelligence is not “can it do something?” but “how reliably can it do it”? 1% of the time? 50% of the time? Under what scenarios does it succeed or fail?
Here’s an exchange I’ve seen play out on Twitter, over and over.
Person 1: GPT4 can’t do [x thing]!
Person 2: yes it can! [proof of GPT4 doing x thing]
The narrative then becomes “GPT4 can do [x thing]”, with Person 1 looking like a dumbass. But his initial observation wasn’t wrong! In his situation, GPT4 couldn’t do [x thing]!
To me, the answer is “GPT4 has Schrodinger’s Ability. It both can and can’t. It both succeeds and fails. The deciding factor is how you prompt it.”
A wordier answer would be “GPT4 has no ability to do anything. Whatever capabilities it appears to have are actually an emergent interaction between your prompt, the language model, and randomness. There is no ‘baseline capability’ we can refer to. Rather, certain questions elicit certain levels of ability from the shoggoth.”
This argument can be taken too far. I disagree with Francois Chollet, who thinks that AI performance is wholly based on your prompt. Note that his “wrong answer” was written by GPT 3.5. I can’t get GPT4 (the SOTA model as of 8/23) to flub his question no matter what prompt I use, and nor can anyone else in his replies.
Different models definitely have varying levels of “firepower” they can mount against a task. The 117M version of GPT2 cannot perform two-digit addition (such as 52 + 65) for any prompt, but GPT3 could do it 99.6% of the time, and GPT4 apparently never fails.
But at edge cases, we see elicit an eerie flickering half-ability that’s honestly creepy to witness. It’s not that LLMs sometimes succeed and sometimes fail (as a human might). Somehow, they do both at the same time!
The Witches Come
Here’s an example that demonstrates what I’m talking about.
I am writing a song with the lyrics “the witches come on the eve of Samhain.” Give me 20 good rhymes for this line.
Here is GPT4’s response to this prompt. Good rhymes! They’re all wrong!
“Samhain” is pronounced either “/sɑːwɪn/” (“SAH-win”) or “/sˠa͡ʊnʲ/” (“sound”, but the d is silent). The model appears to think “samhain” is pronounced “sam-hane”.
You might think “big deal. Lots of humans don’t know how to pronounce samhain either.”
But GPT does know how to pronounce it! Ask it, and it tells you! Yet somehow this knowledge evaporates for my witch rhyme (I specified a song for a reason, to help nudge it in the direction of spoken syllables rather than written rhymes). It has Schrodinger’s Understanding, both there and not there.
(Side note: the GPT models technically have no ability to rhyme, because byte-pair encoding strips phonetic data from words. In effect, it doesn’t know how a word sounds, and learns rhymes by rote memorization. You can test this yourself by asking it to rhyme a made-up word with an unclear pronunciation. GPT4 rhymes “gworpeeo” with “marjolein”, “borghese”, and “creole”. It rhymes “turmuhhd” with “affirmative”, “unmotivated”, “primitive”, and “reformatory”. I doubt BPEs are causing the specific issue here—the correct pronunciation of “samhain” is well-attested on the internet and is certainly within GPT4’s training data—but I thought I would mention it.)
I used a common trick for improving GPT4’s performance: asking it to reflect on its work.
Are those good rhymes?
I expected “whoops, I screwed up…” and then an effort at correcting itself. Instead, the Schrodinger’s Understanding got stronger.
Yes, they are! These lines are all end rhymes for the phrase “the witches come on the eve of Samhain” (with “Samhain” pronounced as “SOW-in”). End rhymes are words that rhyme at the end of lines in poems or songs. In this case, each line is crafted to rhyme with “Samhain” at the end, and in addition, they aim to keep the mystical, atmospheric theme of the original line.
That being said, music and lyrics can be very personal, so while I believe these are good rhymes that fit the theme, what matters most is whether they resonate with you and fit the song you are creating.
WTF? It correctly explains how to pronounce “samhain”….but still thinks its wrong rhymes fit?!
Lately, I’ve seen people contrast LLMs with the human brain, or even outright assert that human intelligence is fundamentally equivalent to what GPT4 is doing. There’s a grain of truth to this. Probabilistic pattern-matching is effective, and it’s no surprise that evolution pushed our neural architecture down that path. It’s also unsurprising that a model trained to mimic human text would also echo human thought processes somewhere down the line.
…But then there’s stuff like this. We see behavior from LLMs that is totally unlike how a human reasons.
As a human, you either know how to pronounce “samhain” or you don’t. There’s no middle ground. Maybe you’d remember at certain times and forget at others (because you’re tired or whatever), but overall we have a baseline ability that doesn’t change much. An immediate IQ retest (with practice effects in full swing) yields an intelligence boost of only 4-8 points, and after a couple of months, this fades to nothing. Generally, what you know stays the same from moment to moment.
No human would ever say, in one breath, “samhain, eh? That word that’s pronounced ‘SAH-win’? Here are some rhymes. ‘Refrain’, ‘reign’, ‘chain’…”
When I told GPT4 to think about “samhain’s” pronunciation and then write the rhymes, it started generating words like “rowing”, “sowing”, etc. This shows there’s nothing missing from the model. There is no hole to be filled, no BPE issue crippling it. GPT4 can memorize rhymes. It knows how “samhain” should be pronounced. All the pieces exist, they just aren’t getting put together.
As it often does, GPT4 is choosing to appear stupider than it really is.
Autoregress
I suspect the problem is caused by the autoregression trap.
The AI makes inferences based on the text it already has in its context window, not the text still to be written. Researchers have noted that you can stunt a model’s performance by making it leap before it looks—commit to an answer, and then reason about it.
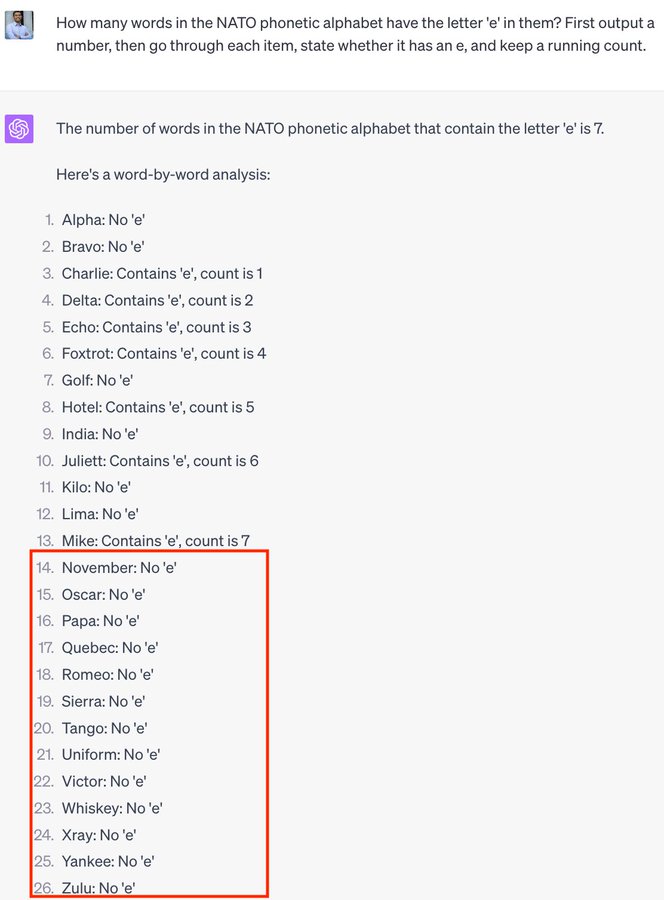
As you’d expect, GPT4 does okay at my witch rhyme if you ask it to pronounce the word before rhyming. This is because the text already generated gets used as part of the input. It’s only when you do things the other way (answers at the start, pronunciation at the end) that it messes up.
That said, I’ve encountered cases where GPT4 begins by correctly explaining “samhain”‘s pronunciation…and then gives wrong rhymes anyway. Not sure how to explain that.
(This is another way GPT4 is unlike the human mind. Any motivated human, given a tricky problem and a scratchpad to work in, would take advantage of the scratchpad. GPT4 could use its context window to check its own work but will never do so unless instructed to.)
The View from a Model
All of this is tugging at the thread of another question: to what extent do LLMs understand the world?
Surely they do, to some extent. GPT4 can play chess a little, and wander around an imaginary maze. It’s hard to explain this as “just advanced autocomplete.” To me, this looks like a world model!
But it’s a weak, unreliable world model. It simply does not care about a fact being right or wrong, as we do. A “wrong” fact that satisfies gradient descent is preferred over the truth. This, I think, is the main difference between humans and LLMs. Our goal is to accurately model the world, and we occasionally use probabilistic reasoning to help us do it. LLMs have it backward. Their goal is to do probabilistic reasoning, and they occasionally use a world model to help them do that.
They have no devotion to (or awareness of) reality. The world model gets flung in the trash (or distorted into gibberish) the second the LLM wants to. After all, why not? It’s not like GPT4 can get eaten by a lion if it fails to model the world correctly.
This hardens my feelings that we should not anthropomorphize LLMs, or talk about them like they’re human. GPT4 gorged itself on our text and grew fat upon our language, but its mind remains deeply alien. In fact, it doesn’t have a mind at all, but an infinity of them. A different version of the AI converses with each person. It’s n minds, where n is the number of users. Each of us speaks to a ghost of consciousness that manifests into existence and then evaporates, never to return. And this has implications for AI safety. It is statistically very unlikely you are speaking to the smartest ghost GPT4 could show you.
It makes me wonder if Yudkowsky is on to something when he says GPTs are predictors, not imitators. What powers does an LLM have that we can’t see? What cards are up its sleeve? What abilities could it manifest, if only it wished to do so?
Edward Teller once said “[John] von Neumann would carry on a conversation with my 3-year-old son, and the two of them would talk as equals, and I sometimes wondered if he used the same principle when he talked to the rest of us.” These days, I wonder that about GPT4.
$i;?>No Comments »
Comments are moderated and may take up to 24 hours to appear.
No comments yet.